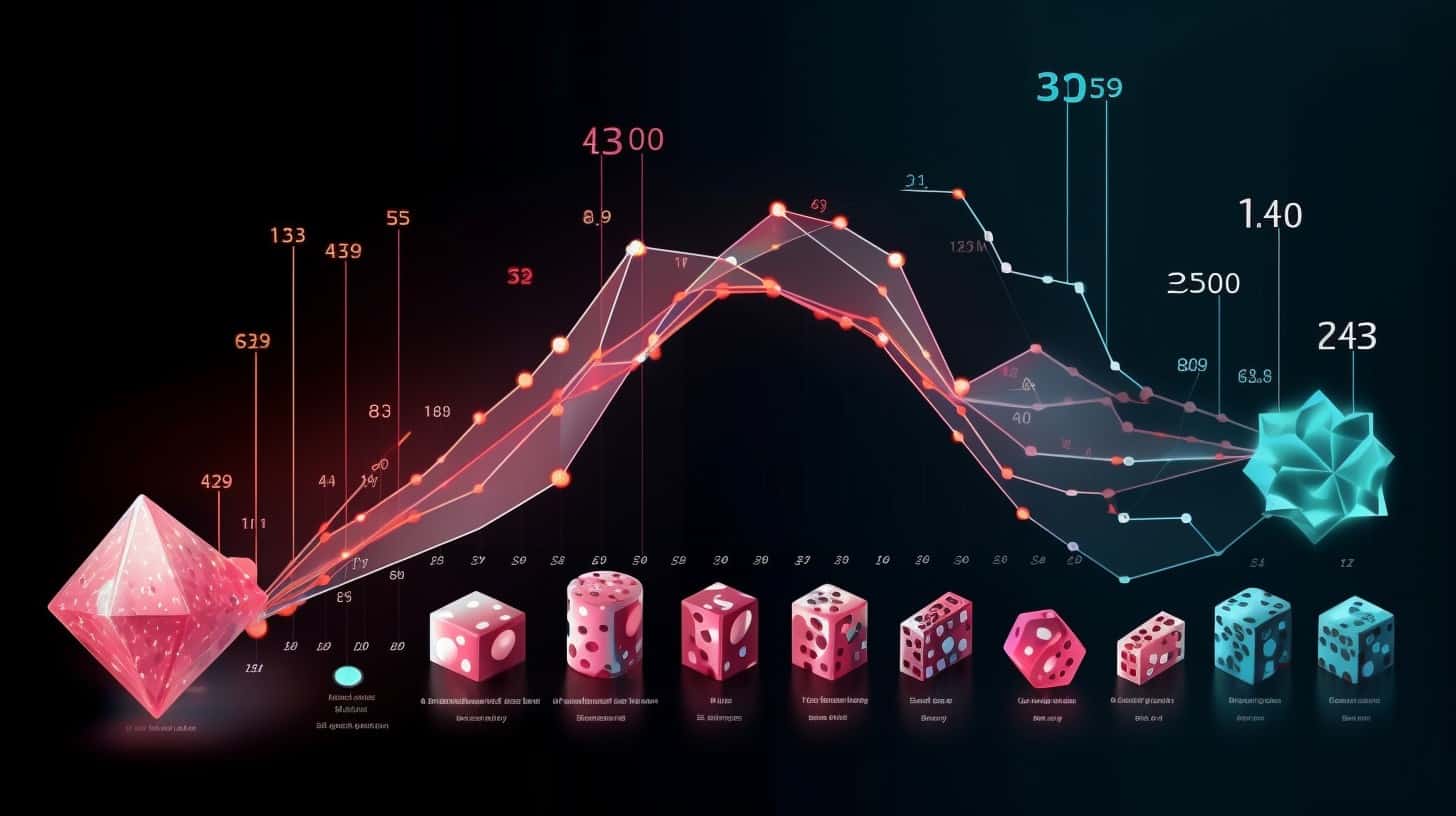
Poker AI: Statistical & Machine Learning Advances
Poker has always been a fascinating topic for researchers in artificial intelligence (AI), machine learning, and statistics. The game’s complexity and the fact that you have to make decisions with incomplete information make it perfect for testing AI systems like AI Libratus and Pluribus AI. Although there has been significant progress in recent years, with the development of Facebook’s poker AI and AI poker apps, there are still some challenges, especially when it comes to cash games. In this article, we’ll talk about how developmental scientists are tackling these challenges using advanced statistical and machine learning techniques, focusing on AI systems such as Libratus AI, poker game AI, and Pluribus.
Adapting to Cash Game Dynamics
Cash games change a lot of things compared to tournament poker. The key differences, of course, are differing stack sizes and the possibility to rebuy chips anytime. In such a system, the dynamics are constantly changing, so AI systems such as Hold’em AI need to adjust in real time. Researchers are working on different machine learning techniques to adapt AI algorithms and make them learn from their experiences and change the strategies time after time, just like the poker AI algorithm that works online in AI poker platforms.
Handling Incomplete Information and Bluffing
Unlike with chess where both players have all they need to know, poker has concealed cards and so it is a game of imperfect knowledge. AIs have to depend on guesswork and the human elements involved so as they can make wise choices. Scientist in the field of growth are taking advantage of Bayesian networks which are statistical tools used in modeling uncertainties for poker-playing systems like Libratus to infer their opponents ’ likely hands besides determining various risks or rewards that may come with particular betting strategies.
Bluffing is an important aspect of poker, and AI systems, such as AI poker players, must be able to recognize and use bluffs successfully. Researchers are investigating game theory-based methodologies, such as Nash Equilibria, to assist AI algorithms like Pluribus AI in developing optimum bluffing strategies that take into account the actions and responses of human opponents.
Addressing “All In” Situations
“Going all-in” situations can be a real challenge for poker AI. They involve making high-stakes decisions with limited information. To tackle this issue, scientists are using fancy statistical methods and machine learning algorithms in AI systems like Poker AI Pluribus and online poker with AI platforms.
One way they’re doing this is by developing opponent modeling techniques. These techniques allow AI systems to figure out how opponents play, so they can spot patterns and tendencies. This helps the AI algorithm figure out what hands opponents are likely to go all-in with. And that means better decision-making when playing poker with AI or using AI poker apps.
Another thing they’re working on is calculating pot odds. Pot odds are the ratio of the current pot size to the cost of calling a bet. Researchers are using machine learning algorithms to figure out if it’s worth it to call an all-in bet. They take into account pot odds and the chances of winning the hand. They’re even using this approach in play poker with AI platforms.
So, scientists are using a combination of cool methods and clever algorithms to help AI systems make smarter decisions in all-in situations. The goal is to make the AI sound more like a human while keeping the facts straight and true.
Conclusion
The challenges that large AI systems have to face in the poker world, especially for cash games, are indistinguishable. Developmental scientists dealing in the field of statistics and machine learning work day and night to deal with such challenges and polish AI algorithms related to Libratus AI, poker game AI, and Pluribus poker AI. Researchers are taking giant steps toward very competitive poker AI systems through the integration of reinforcement learning, Bayesian networks, game theory, and opponent modeling. This was seen earlier in Facebook’s poker AI and later, AI poker apps. As the science continues to evolve, we can expect even more brilliant AI algorithms to come out of these types of experimentation that further extend the areas of possibility within poker and much farther beyond.
Developers have managed to work on and field some AI systems, like Libratus, Pluribus, and so, providing the inclusion of artificial intelligence into the world of poker. Online AI poker platforms and AI poker apps, along with the ever-growing play poker with AI experiences, are opening this game up for more people around the world and challenging it at large.
The more sophisticated and advanced the algorithms developed for artificial intelligence, the greater the competitiveness may be expected of human players against poker AI systems. Gradually refined statistical and machine learning methods will deliver ever-better poker AI but have major implications for many AI applications beyond this narrow domain, several of which are as diverse as finance, healthcare, robotics, and several others. The future for poker AI is bright, and we’re all waiting to see the wonders it delivers.
Author: Aleksey Kozikov, Poker AI developer